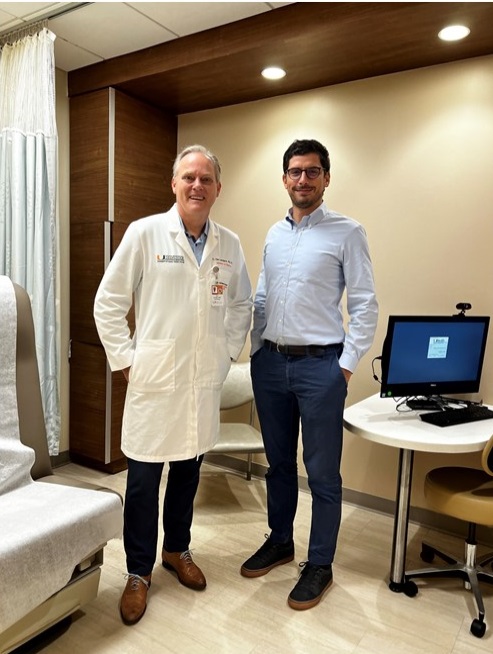
In recent years, there has been remarkable progress in enhancing the clinical outcomes of multiple myeloma (MM) through the development of innovative therapies. Despite the availability of newer and more effective treatments, some newly diagnosed MM (NDMM) patients still face poor clinical outcomes. Unfortunately, existing prognostic models struggle to accurately predict the individual patient’s clinical outcomes in relation to a given therapy, hindering the development of personalized strategies. Existing prognostic models are designed to provide the average clinical outcomes for all patients treated with a specific therapy. However, if a patient asks, “given my disease biology and my clinical status, what is my predicted progression-free survival (PFS) if I were to be treated with therapy XYZ?” To date, no model has been able to predict the best answer based on available data. To improve models to overcome these challenges, investigators are using artificial intelligence to analyze multiple complex data sets.
Through a collaborative effort, including Memorial Sloan Kettering Cancer Center, NYU Langone Health, Moffitt Cancer Center, Heidelberg University Hospital, the Multiple Myeloma Research Foundation, and Sylvester Comprehensive Cancer Center at the University of Miami, investigators recently published results (Journal of Clinical Oncology) compiled from a database of 1933 NDMM patients, incorporating clinical and genomic information. The study introduced a novel genomic classification that builds upon previous models based on gene expression and fluorescence in situ hybridization (FISH). The investigators observed two mutually exclusive patterns: one characterized by complex genomic events and aneuploidies, and the other displaying an indolent nature rarely affected by major genomic events. This classification, incorporating novel genomic events, not only enhances the understanding of MM biology but also lays the foundation for future targeted and genomic-oriented therapies. Additionally, the investigators developed a deep learning model called Individualized Risk in NDMM (IRMMa). This model introduced key innovations: (i) It corrects for time-dependent features, including high-dose melphalan followed by autologous stem cell transplantation (HDM-ASCT) and continuous treatment (maintenance). (ii) Also, IRMMa integrates genomic features and considers 32 different treatment combinations, primarily involving proteasome inhibitors and immunomodulatory drugs (IMIDs). Notably, thanks to its design and innovations, IRMMa demonstrated significantly higher accuracy compared to traditional prognostic models such as ISS, R-ISS, and R2-ISS. Its performance was also validated on an independent dataset from Heidelberg University (clinical trial GMMG-HD6). (iii) Finally, IRMMa successfully predicted cases where HDM-ASCT provided an advantage and others where it did not impact outcomes.
Based on these recent results, IRMMa represents an innovative opportunity to explore the heterogeneity of NDMM patients, addressing the oversimplified current understanding. The model not only improves our comprehension of outcomes in both past and future clinical trials but also opens avenues for refining personalized treatment strategies in multiple myeloma. IRMMa represents is a major step in the direction of precision medicine for patients with multiple myeloma.
Collaboration and Contributions:
- Sylvester Comprehensive Cancer Center at the University of Miami
- Moffitt Cancer Center for the AVATAR data.
- MSKCC for the MyType data
- NYU Langone Health and UAMS for their targeted data.
- NYU Langone Health and MRC UK myeloma groups for the MRC XI
- Heidelberg for the GMMG-HD6 data
- MMRF with the CoMMpass data